Sitting In A Room: Celebrating The Sound of AI's Mistakes
As AI learns from AI, what new sounds are waiting to be discovered?
Hello, hello – good.
Sorry, this is a little late. Last week was wild with new projects as well as hitting the road to do a keynote at Tomorrowland Academy. It’s a new concept taking place the week between the two festival weekends in Boom, Belgium, with DJ and music production lessons, guest speakers and talks from bookers, agents, producers, DJs and other people from the industry.
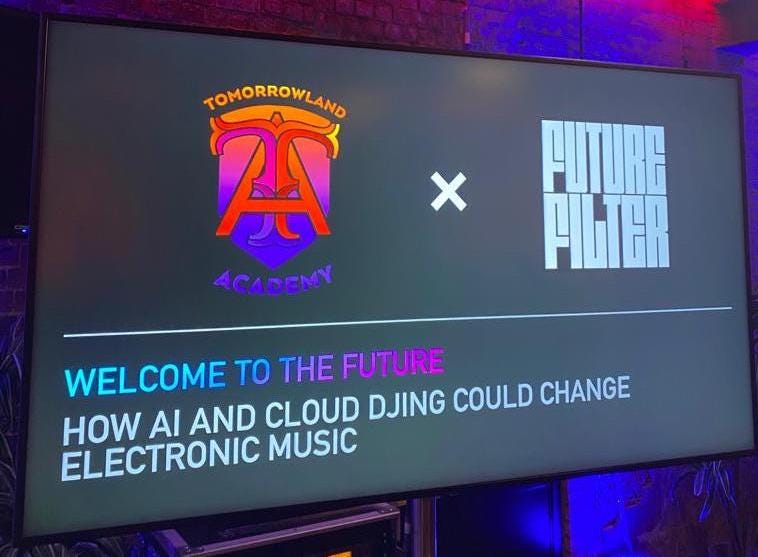
They asked me to give an overview of the AI and Cloud DJing landscape, which was good as it’s exactly what I’ve been writing about on Future Filter for the past four weeks.
It was really interesting to get a reading outside the tech industry bubble and endless hype and doom to see what young producers actually cared about when it came to AI and how it might affect their music-making processes.
More on that another time.
For now, a quick experiment that turned out to be pretty fun and a really stark example of how quickly things could turn interesting when AI starts to eat itself.
Declan x
The Week in AI
Daniel Ek is excited about how AI and AR can be used for advertising on Spotify, as well as improved discovery and other features. He made the comments on their latest earnings report call.
As I was writing this, the excellent newsletter Music x published a similar piece on synthetic data and art, which is a must-read.
Spawning.ai – a website that lets rightsholders opt out of AI training – has introduced a new Chrome browser extension that lets you scan the current page for images used in public datasets.
BTS’s label and 360 music company HYBE is developing an AI technology that can translate songs for different markets. The complexity here is deep, with different syllables and intonations across languages making it difficult to align words and melodies. Reuter’s reported on the tech here, and you can hear it in action. In theory, it sounds really impressive, though there are for sure cultural appropriation concerns.
Sitting In A Room
When I was studying Music Technology, one of the pieces that always stuck with me was Alvin Lucier’s ‘I Am Sitting in a Room’. If you’re not familiar with this piece of sound art, Lucier recorded himself saying a few sentences explaining the concept. He then replayed the recording in the same room, using loudspeakers, and recorded the speaker output using the same mic. He then did this again, and again, and again.
Eventually – and actually much quicker than you might think – the resonances of the room start to dominate until his original voice disappears completely into a smear of bubbling frequencies that sound like some kind of haunted echo. It’s also very beautiful and sounds entirely musical despite the original source being a monotonous human voice.
Every room has a resonance, and every sound has a fundamental frequency and harmonics that give it its characteristics. By recording the same audio over and over, Lucier was able to turn up the ‘room’ and turn down the ‘source’, lifting the curtain on the subtle sounds that make up our world. It always made me wonder what other secret sounds are occurring in the form of echos, nodes and resonances and how they affect our perceptions day to day.
It made me think, too, about how much work we put into removing those ‘mistakes’ when recording music. Nodes, resonances, standing waves and slapback are considered bad things, and recording studios spend tens of thousands of pounds making sure they don’t make it into the final take. I’m not saying that’s the wrong approach, I just always thought it was interesting how these ‘mistakes’ in Lucier’s piece eventually became something very otherworldly and musical in their own right. There’s beauty hidden in those sounds.
It always made me wonder what other secret sounds are occurring in the form of echos, nodes and resonances and how they affect our perceptions day to day.
Codec Moment
41 years after Lucier’s original piece, YouTuber Ontologist replicated the concept. Instead of re-playing audio back into a space to accumulate resonance, he uploaded a video to YouTube, downloaded that video and re-uploaded it, over and over until he reached 1,000 uploads. The result is a gradual decrease in quality as artefacts from both YouTube’s compression and the MP4 codec are introduced, eventually resulting in a smear of white visuals and watered-down audio that again, sounds alien and otherworldly. You can watch it below.
The difference between Ontologist’s experiment versus Lucier's is that the 1969 piece highlights a wholly natural phenomenon that occurs in every space where sound is present (apart from an anechoic chamber). Ontologist on the other hand introduced a newer phenomenon in the form of digital compression to the story. What’s interesting is that both, although completely unrelated and opposite in their origins, result in a similar, almost ethereal output, where the ‘mistakes’, once multiplied, take on a life of their own.
AI Echo
If you’ve made it this far, you might wonder where I’m going with this. While I was travelling back from Brussels this week, I found myself reading a few articles about AI chatbots ‘eating themselves’.
When models like ChatGPT first appeared, they were trained on ‘the internet’, warts and all. There were of course mistakes and errors strewn throughout that data, as there has been on the internet since its inception, but they were human-made mistakes.
One article in particular – The Atlantics’ ‘AI Is an Existential Threat to Itself’ – throws up the idea: What happens when the internet starts to be populated with AI-generated content, and the next iteration of LLMs are trained on that version of the internet? And then, more content is generated by chatbots, more LLMs are trained on that content and so on.
In the piece, Matteo Wong writes about a language model that was tested:
“The program at first fluently finished a sentence about English Gothic architecture, but after nine generations of learning from AI-generated data, it responded to the same prompt by spewing gibberish: ‘architecture. In addition to being home to some of the world’s largest populations of black @-@ tailed jackrabbits, white @-@ tailed jackrabbits, blue @-@ tailed jackrabbits, red @-@ tailed jackrabbits, yellow @-.’”
It’s not just chatbots and text models – image generators too will be retrained on their own outputs, and given the often wonky results from DALL-E, Midjourney and others, will training on that data result in an even more warped version of reality staring back at us?
The article quotes sci-fi writer Ted Chiang, who explains if ChatGPT is a condensed version of the internet, akin to how a JPEG file compresses a photograph, then training future chatbots on ChatGPT’s output is “the digital equivalent of repeatedly making photocopies of photocopies in the old days. The image quality only gets worse.”
There are more serious consequences to consider around mistakes and bias in AI systems if they end up being trained on their own outputs. A recent episode of the NY Times podcast Hard Fork discussed some of those here. But for creative purposes, the story is more nuanced.
The same argument could be made that Lucien’s resonances and Ontologist's artefacts are ‘worse’ representations of the original sources. Technically that’s true, but the end results of both are totally unique and for sure have artistic merit.
I found myself wondering: ‘What happens when we train an AI voice model on AI-generated voices?’ What might it sound like after, 10, 20 or 100 iterations? What kind of unique sounds might leak in as the AI attempts to transfer one style to another, over and over again? I decided to find out.
Training future chatbots on ChatGPT’s output is “the digital equivalent of repeatedly making photocopies of photocopies in the old days. The image quality only gets worse.”
An Experiment
Kits.ai is an AI voice modelling marketplace that offers both royalty-free voices to use with your own acapella, or paywalled voice models with more established singers and artists. I wrote about them a few weeks ago in my ‘How Do You Build an AI Voice Model Marketplace?’ newsletter. Users upload as high-quality an acapella as they can and choose which singer they want to transfer the style from a drop-down list.
I decided to experiment by recording myself repeating a Lucier-style sentence (although much shorter to quicken the conversion time), using it as an input for a model, downloading the output and re-uploading that as the next input, to see what kind of output we’d get after 10 uploads. I could have gone to 100, but I think you get the idea pretty quick.
The result was kind of wild although I should point out, these models are designed to be used with singing voices, not with spoken word, so it wasn’t a true representation. And also, I used their browser recording feature for the original file, so I don’t have that recording! Only the first output. (If anyone knows if I can extract that cached recording from somewhere deep inside my browser, let me know).
Here’s the first output and the 10th output (sadly, I can’t embed playlists on Substack but you can click through to hear all 10 outputs individually). I don’t actually sound like this btw, you’ll have to subscribe and DM for a private voice reveal.
As you can hear, it’s kind of fun, kind of weird and maybe kind of cool? I like the direction of travel, and how quickly it started to break down. Even by the third iteration, it wants to break into song, which is indicative of the model used – a singer called Aariz, hence the name.
Accumulation Emulation
This isn’t a scientific study of AI data pollution, or its impact on humanity, which could genuinely be profound as The Atlantic article points out. This is simply a loose experiment with both Lucien and Ontologist in mind. Both flipped the narrative on what ‘mistakes’ could be.
It seems to me that music production has moved towards degradation, attempting to add VHS effects, a lust for old samplers and their 12-bit sound and re-introducing imperfection to a precise digital environment. Not just in music but in photography, film and beyond. The search for perfection has left us cold – something we discussed at length in last week’s newsletter.
Where some might (rightly) laugh at AI’s failings when it comes to sample-accurate, lossless reproduction of studio-quality music, I see this period as a creative opportunity. If early digital samplers could have been perfect, they would have been. But by falling short, they introduced their own kind of beauty that has become a sought-after sound. Similar to the TR-808 and the 909’s failings when it comes to ‘real’ drum replication and the 303’s failed attempt at being a bass line accompanier for organists, limitations tend to bring out the best in creativity and can even usher in new genres and styles.
Not every technically deficient sound is remembered fondly – few would pang for the thin sound of early VSTs, for example. Will we see the ‘early sound of AI’ become a longed-for aesthetic in years to come? I’m not sure, but with Lucier’s piece in mind, there are often new, unexplored sounds and tones to be found within these ‘mistakes’. In the space between flaw and perfection, there’s some magic to be found.
Try it yourself, Kits.ai is free and you can check their site here.
Recommended Reading
AI Is An Existential Threat To Itself (The Atlantic, 2023)
The AI feedback loop: Researchers warn of ‘model collapse’ as AI trains on AI-generated content (VentureBeat, 2023)
Is AI Poisoning Itself? (Hard Fork, 2023)
Other recommended stuff from the internet
DVS1’s Aslice – a platform where DJs can pay a percentage of their fee to the producers who make the music they play in that set – has announced its first PRO partnership. Canada’s SOCAN will now accept data from Aslice’s playlist info, helping to close any data gaps that might exist on their end and make sure artists are paid promptly and accurately. Love to see it. Create Digital Music wrote about it here. Here’s to many more PROs getting on board.
Spotify has raised its prices globally which SHOULD mean more money for artists given that part of their payouts are linked to revenue share.
Wow, the difference between analogue and digital disintegration is stark here, between the original and the YouTube version. Great piece!